Unlocking Success with Open Source Image Annotation Tools
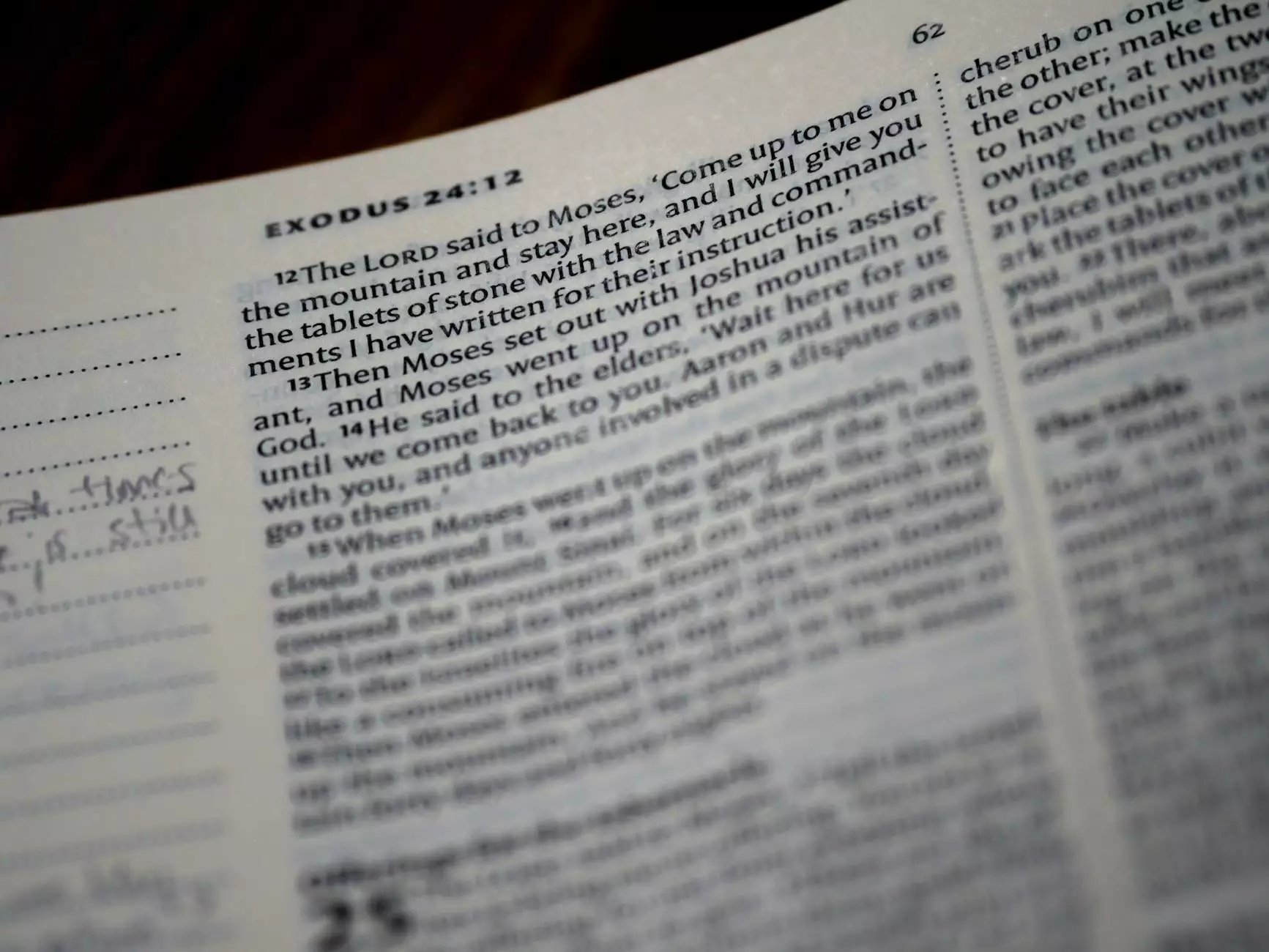
Image annotation tools play an essential role in data preprocessing and machine learning model development, especially in computer vision. These tools are crucial for labeling data accurately, which is a significant step in training artificial intelligence (AI) algorithms. In this comprehensive article, we will explore the world of image annotation tool open source options available to businesses, including their benefits, features, and how they can impact your data annotation platform.
What is Image Annotation?
Image annotation involves the process of identifying and labeling objects within images. This technique is vital for creating datasets that can be used in training machine learning models. With the right tools, businesses can effectively annotate images, enhancing the accuracy and efficiency of their AI models.
Benefits of Using Open Source Image Annotation Tools
- Cost-Effective: Open source solutions usually come at no cost, allowing businesses to save on licensing fees while accessing high-quality tools.
- Customization: These tools can often be tailored to meet specific needs, making them ideal for various industries.
- Community Support: A vibrant community of developers and users provides support, updates, and improvements constantly.
- Transparency: With open source, businesses can review the code for security purposes and better understand how the tool operates.
- Integration: Many open-source tools allow easy integration with existing systems, including popular data annotation platforms.
Key Features of Effective Image Annotation Tools
When evaluating image annotation tools, businesses should look for several key features to ensure they select the right option for their needs:
1. User-Friendly Interface
An intuitive interface is crucial for minimizing the training time for new users. Tools that allow users to navigate easily and annotate images efficiently can lead to better productivity.
2. Multiple Annotation Types
Different projects may require various types of annotations. Ensure the tool supports polygon, bounding box, keypoint, and semantic segmentation annotations to accommodate diverse requirements.
3. Collaboration Capabilities
Effective teamwork improves annotation productivity. Look for tools that allow multiple users to work simultaneously, track progress, and communicate efficiently.
4. Export Options
Flexibility in exporting data is essential to integrate with different machine learning frameworks. The ability to export in popular formats like COCO, Pascal VOC, and YOLO is particularly beneficial.
Top Open Source Image Annotation Tools
1. LabelImg
LabelImg is a popular desktop application that allows for quick and easy image annotations using bounding boxes. It's user-friendly and provides a straightforward path for exporting annotations in Pascal VOC and YOLO formats.
2. VGG Image Annotator (VIA)
The VGG Image Annotator is a simple and effective tool developed by the Visual Geometry Group at Oxford University. It supports various annotation types including point, bounding box, and polygon, making it versatile for many annotation tasks.
3. RectLabel
RectLabel is an annotation tool specifically designed for macOS users. It supports labeling images for object detection and segmentation tasks. Users appreciate its ease of use and quick export capabilities to popular formats.
4. CVAT (Computer Vision Annotation Tool)
CVAT is a powerful tool developed by Intel. It provides advanced features like interpolation for video annotations, automatic annotation capabilities, and extensive labeling options suitable for professional projects.
5. Supervisely
Supervisely is another comprehensive platform offering annotation and management capabilities, perfect for teams working on extensive image datasets. It integrates well with deep learning frameworks, offering seamless workflows.
Integrating Open Source Annotation Tools with Your Data Annotation Platform
Integrating open source tools into your existing data annotation platform can enhance your overall workflow. This integration can optimize resource utilization, improve accuracy, and increase the speed of data preparation.
Here are some strategies for effective integration:
1. API Utilization
Many platforms offer APIs that facilitate the integration of annotation tools. Utilize these APIs to connect your chosen open source tool with your existing systems for automatic data flow.
2. Workflow Automation
Automate repetitive tasks in your workflow to improve efficiency. For instance, combine multiple tools to handle different parts of the workflow seamlessly.
3. Training and Onboarding
Ensure all team members are adequately trained on using the integrated tools. Providing comprehensive training reduces the learning curve and enhances productivity.
Challenges in Image Annotation and How to Overcome Them
While image annotation tools, especially open source ones, offer numerous advantages, challenges can arise. Here are common issues and strategies to overcome them:
1. Quality Control
Ensuring the quality of annotations is vital for model accuracy. Implement a review system where annotations are verified by multiple team members to maintain high standards.
2. Scalability
As projects grow, handling larger datasets can become overwhelming. Solutions include using batch processing to manage multiple images and employing automated tools to assist in the annotation process.
3. Task Management
Managing tasks effectively is crucial, particularly in larger teams. Use project management tools to assign tasks, track progress, and obtain feedback from team members.
Future Trends in Image Annotation
As the field of machine learning continues to evolve, so too does the technology behind image annotation. Here are some trends to watch:
1. Automation and AI-Assisted Annotation
With advancements in AI, many image annotation tools now include features for automatic labeling, reducing the manual effort required and speeding up the annotation process.
2. Collaborative Annotation Platforms
Future tools will increasingly focus on collaboration, enabling teams to work together in real-time and leverage collective expertise.
3. Enhanced User Experience
As competition grows, tools will continue to prioritize user experience, with more intuitive interfaces and streamlined processes, making image annotation accessible to all.
Conclusion
Choosing the right image annotation tool open source is critical for businesses aiming to enhance their data annotation processes. With a plethora of excellent options available, firms can leverage these tools to drive efficiency and accuracy in their machine learning endeavors. By understanding the benefits, features, and future trends associated with image annotation, companies can better position themselves for success in an ever-evolving technological landscape.
For those interested in leveraging the best data annotation tools available, visit keylabs.ai and discover how we can help your business thrive in today's competitive market.