Malware Detection Using Machine Learning: A Crucial Step for Business Security
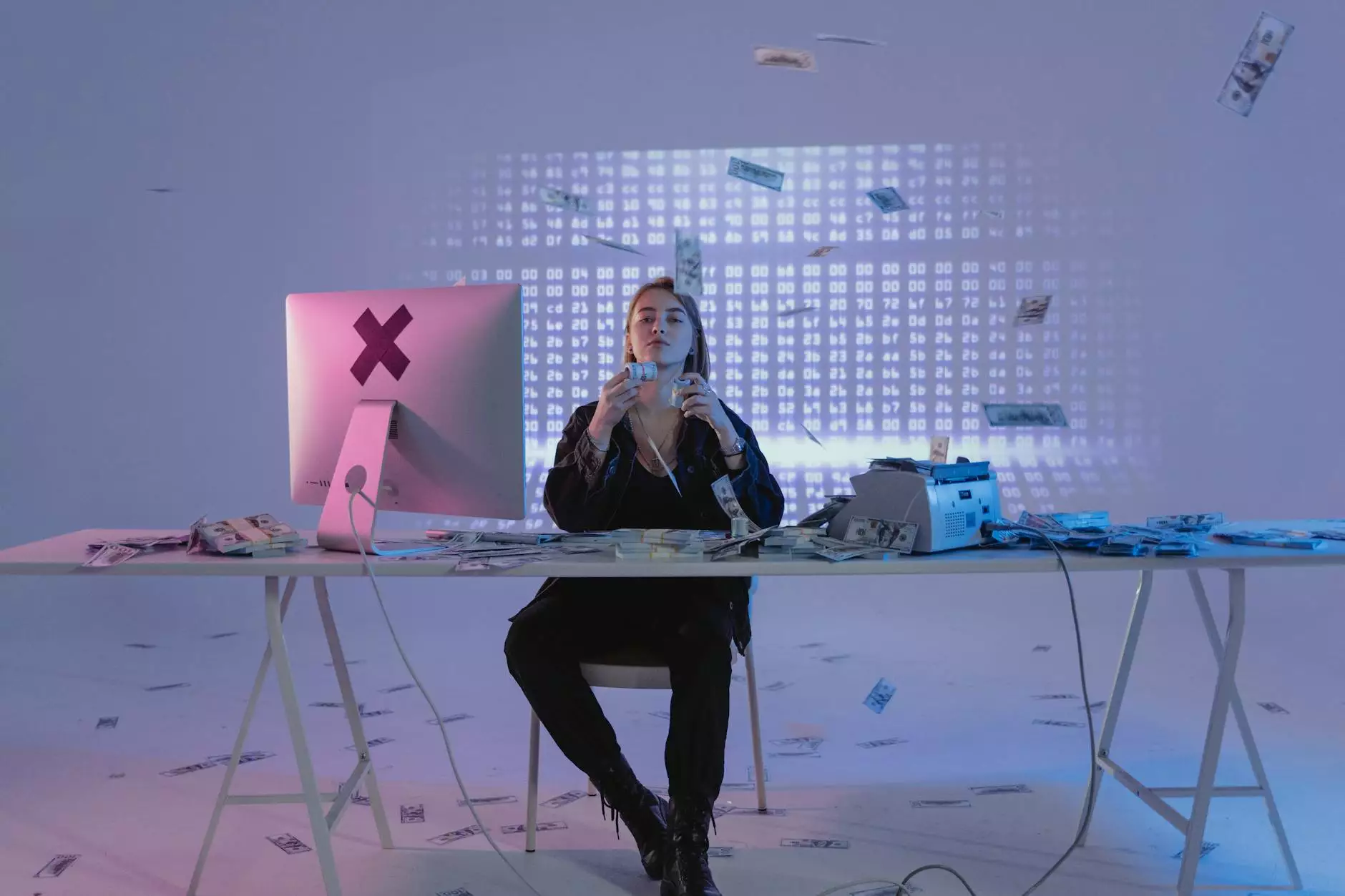
In an increasingly digital world, where businesses rely heavily on technology for their day-to-day operations, the threat of malware has escalated significantly. Malware detection using machine learning has emerged as a powerful method to combat this issue. As organizations embrace more sophisticated technologies, they must also leverage advanced security systems to protect their sensitive data and infrastructure. At Spambrella, we are dedicated to providing top-notch IT services and security systems designed specifically to thwart these evolving threats.
Understanding Malware: What You Need to Know
Before diving into the intricacies of malware detection using machine learning, it's essential to understand what malware is and the various forms it can take. Malware, short for malicious software, is designed to disrupt, damage, or gain unauthorized access to computer systems. The most common forms include:
- Viruses: Malicious code that attaches itself to clean files and spreads throughout a system.
- Worms: Self-replicating malware that spreads across networks without human intervention.
- Trojans: Malicious software disguised as legitimate software.
- Ransomware: A type of malware that locks your files, demanding a ransom for their release.
- Spyware: Software that secretly monitors user activity and collects personal information.
The Limitations of Traditional Malware Detection
Traditional malware detection methods typically rely on known signatures, meaning they can only identify malware that has been encountered before. While effective to some extent, these systems are increasingly inadequate against new and sophisticated threats. Further, as cybercriminals continuously evolve their tactics, relying solely on signature-based detection puts businesses at risk. This is where machine learning comes into play.
How Machine Learning Enhances Malware Detection
Machine learning (ML) refers to algorithms that enable systems to learn and improve from experience without being explicitly programmed. When applied to malware detection, ML offers several advantages:
- Predictive Analysis: Machine learning models analyze vast amounts of data to predict potential threats based on patterns and behaviors rather than relying on known malware signatures.
- Anomaly Detection: These systems can learn what normal activity looks like in a network. When they detect deviations from these patterns, they can flag it as suspicious behavior that may indicate the presence of malware.
- Real-Time Threat Response: ML algorithms can respond to threats in real-time, significantly reducing the window of vulnerability.
- Continuous Learning: As new malware emerges, these systems can adapt and improve their detection methods without the need for constant manual updates.
Implementation of Machine Learning for Malware Detection
Implementing malware detection using machine learning involves several steps:
1. Data Collection
The first step in implementing machine learning for malware detection is to gather data. This includes:
- Network traffic data
- File properties and metadata
- User behavior patterns
- System logs
By collecting comprehensive data, organizations can train their machine learning models to recognize patterns indicative of malware.
2. Feature Selection
Not all data collected is relevant, so selecting the right features is crucial. Important features may include:
- File size and type
- Creation and modification dates
- Source of the file
- User access patterns
3. Model Training
With the collected data and selected features, organizations can now train their machine learning models. This process involves using algorithms such as:
- Decision Trees
- Random Forests
- Support Vector Machines (SVM)
- Neural Networks
The chosen algorithm will depend on the organization’s specific needs and the complexity of the data.
4. Model Evaluation
After training the model, it must be evaluated to determine its effectiveness. This involves testing it against known malware to assess its accuracy and identifying any false positives or negatives.
5. Deployment and Monitoring
Once the model is trained and evaluated, it's deployed into the business’s operational environment. Continuous monitoring is necessary to ensure its ongoing effectiveness and to accommodate new threats.
Benefits of Malware Detection Using Machine Learning for Businesses
The benefits of adopting malware detection using machine learning extend beyond mere identification of threats:
- Cost Efficiency: By detecting malware early, businesses can avoid costly data breaches and downtime.
- Improved Security Posture: Traditional methods often leave gaps in security; machine learning fills these gaps.
- Enhanced Customer Trust: Keeping customer data secure builds trust and strengthens your brand reputation.
- Scalability: Machine learning systems can be scaled to accommodate growing amounts of data as a business expands.
Challenges in Implementing Machine Learning for Malware Detection
While the benefits are compelling, businesses also face challenges when implementing malware detection using machine learning:
- Data Privacy: Collecting sufficient data for training must be balanced with respecting user privacy.
- Resource Intensive: Developing and maintaining machine learning systems can be resource-heavy in terms of both time and finances.
- Skill Gap: There may be a lack of qualified personnel to develop and implement these advanced systems.
Best Practices for Businesses Using Machine Learning in Malware Detection
To ensure the successful implementation of malware detection using machine learning, businesses should consider these best practices:
- Invest in Training: Train your staff in cybersecurity best practices and the basics of machine learning.
- Regular Updates: Keep your machine learning models updated with the latest data to adapt to new threats.
- Integration: Ensure that machine learning systems are integrated with existing security policies and services.
- Incident Response Planning: Have a defined incident response plan in place to act swiftly when malware is detected.
The Future of Malware Detection: The Role of AI
Looking ahead, the landscape of malware detection using machine learning will continue to evolve, especially with the integration of Artificial Intelligence (AI). AI's ability to analyze data at an unprecedented scale will further enhance detection capabilities, leading to:
- Greater Accuracy: AI and machine learning can vastly improve detection accuracy, dramatically reducing false positives.
- Autonomous Response: Future systems may autonomously initiate responses to detected threats, minimizing the need for human intervention.
- Adaptive Learning: AI can continue to learn from newly identified threats, evolving its methodologies in real-time.
Conclusion: The Imperative of Adopting Advanced Malware Detection Techniques
In summary, malware detection using machine learning is no longer just an option for businesses; it is a necessity in today’s digital landscape. The prevalence of cyber threats requires organizations to adopt advanced techniques to safeguard their data and systems. Spambrella is committed to integrating innovative IT services and security systems that empower businesses to thrive in a secure environment. By investing in modern malware detection strategies and leveraging the capabilities of machine learning, businesses can protect themselves against the increasing wave of cyber threats.