The Significance of Agriculture Datasets for Machine Learning
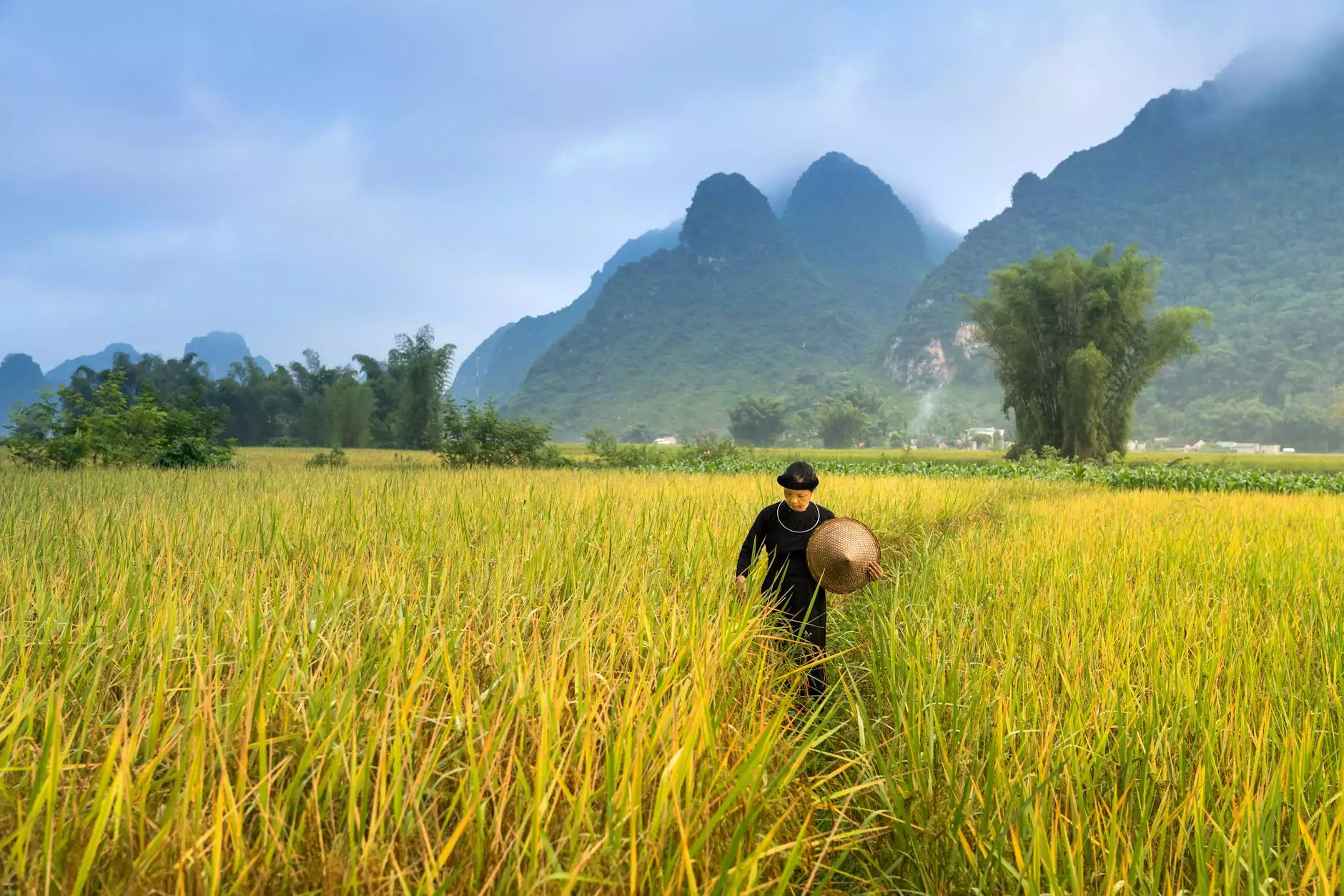
In the age of technology, the intersection between agriculture and machine learning is transforming the way we approach food production, resource management, and sustainability. With the exponential growth of data in the agricultural sector, the use of an agriculture dataset for machine learning has become pivotal in driving innovation and efficiency. This article delves deep into the unparalleled benefits, applications, and future potential of these datasets in reshaping agriculture.
Understanding Agriculture Datasets
To fully appreciate the role of agriculture datasets in machine learning, it's crucial to first understand what constitutes these datasets. Generally, agriculture datasets encompass a wide variety of information derived from various sources, including:
- Soil data – pH levels, nutrient content, moisture levels
- Climate data – temperature, precipitation patterns, humidity
- Crop yield data – historical crop yields, harvest timing, and expected outputs
- Pest and disease data – incidence rates, risk factors, and mitigation measures
- Market data – pricing trends, market demand, and consumer preferences
Why Use Agriculture Datasets for Machine Learning?
The incorporation of machine learning with agriculture datasets enables farmers and agribusinesses to make data-driven decisions that enhance productivity and sustainability. Here are some compelling reasons why these datasets are indispensable:
1. Improved Yield Prediction
One of the most crucial applications of an agriculture dataset for machine learning is yield prediction. By analyzing historical yield data alongside environmental variables, machine learning models can predict future yield outcomes with remarkable accuracy. This predictive capability allows farmers to:
- Make informed planting decisions
- Optimize resource allocation
- Plan for market fluctuations
2. Enhanced Crop Management Practices
Machine learning helps in understanding the complex interplay between soil properties, weather conditions, and crop health. By utilizing these datasets, farmers can:
- Determine optimal planting times
- Identifying the right crop varieties for specific environments
- Monitor crop health effectively using remote sensing technologies
3. Sustainable Farming Solutions
With the increasing awareness of environmental issues, leveraging agriculture datasets for machine learning encourages sustainable farming practices. For example, precision agriculture techniques use data to reduce the quantity of inputs like water and fertilizers, minimizing environmental impact while maximizing productivity.
Machine Learning Algorithms in Agriculture
The success of using an agriculture dataset for machine learning largely depends on the algorithms employed. Here are some commonly used algorithms in this domain:
1. Decision Trees
Decision trees are highly interpretable models that help in making decisions based on data. They find patterns in data that relate to crop success or failures.
2. Neural Networks
Deep learning, a subset of neural networks, excels at recognizing patterns in large datasets. It's particularly useful for image classification, helping in detecting plant diseases from images.
3. Support Vector Machines (SVM)
SVMs are powerful for classification problems, such as categorizing crop diseases based on various factors from datasets.
Sources of Agriculture Datasets
For machine learning applications, obtaining high-quality datasets is essential. Here are some reputable sources where you can find agriculture datasets:
- Government Agricultural Departments – Many countries have open data portals where agricultural statistics are published.
- Research Institutions and Universities – Academic research papers often provide datasets collected through studies.
- Open Data Initiatives – Platforms like Kaggle and the UCI Machine Learning Repository offer datasets specifically for machine learning.
- Private Companies – AgTech companies often provide datasets as part of their software offerings.
Challenges in Utilizing Agriculture Datasets
While the advantages of using agriculture datasets for machine learning are undeniable, several challenges still exist:
1. Data Quality
Data must be accurate, relevant, and up to date. Poor quality data can lead to misleading results.
2. Data Integration
Integrating data from various sources can be complex, requiring significant preprocessing efforts to ensure compatibility.
3. Privacy and Ethical Concerns
Handling sensitive agricultural data can raise privacy issues, necessitating compliance with regulations and ethical standards.
Future Trends in Agriculture Machine Learning
Looking forward, the future of agriculture and machine learning holds immense promise. Here are some trends to watch:
1. IoT and Smart Farming
The Internet of Things (IoT) allows for real-time data collection through sensors placed in fields. This technology empowers farmers by providing immediate insights based on comprehensive agriculture datasets.
2. Autonomous Machinery
The rise of autonomous tractors and drones, powered by machine learning algorithms, aims to reduce labor costs and increase efficiency in crop management.
3. Personalized Farming Experiences
Machine learning will enable tailored farming solutions, where recommendations can be made for specific farms based on unique conditions and available datasets, leading to higher yields and reduced resource usage.
Conclusion
In conclusion, the integration of an agriculture dataset for machine learning is revolutionizing the agricultural landscape. By leveraging data-driven insights, farmers and agribusinesses can enhance productivity, adopt sustainable practices, and make informed decisions that lead to improved outcomes. As technology continues to evolve, the fusion of agriculture and machine learning will unlock new frontiers, ensuring food security and sustainability for generations to come.
For those in the software development industry, particularly at keymakr.com, understanding and utilizing these datasets could pave the way for innovative solutions that support the agricultural sector. The possibilities are endless, and the future is bright for those who harness the power of data and machine learning in agriculture.